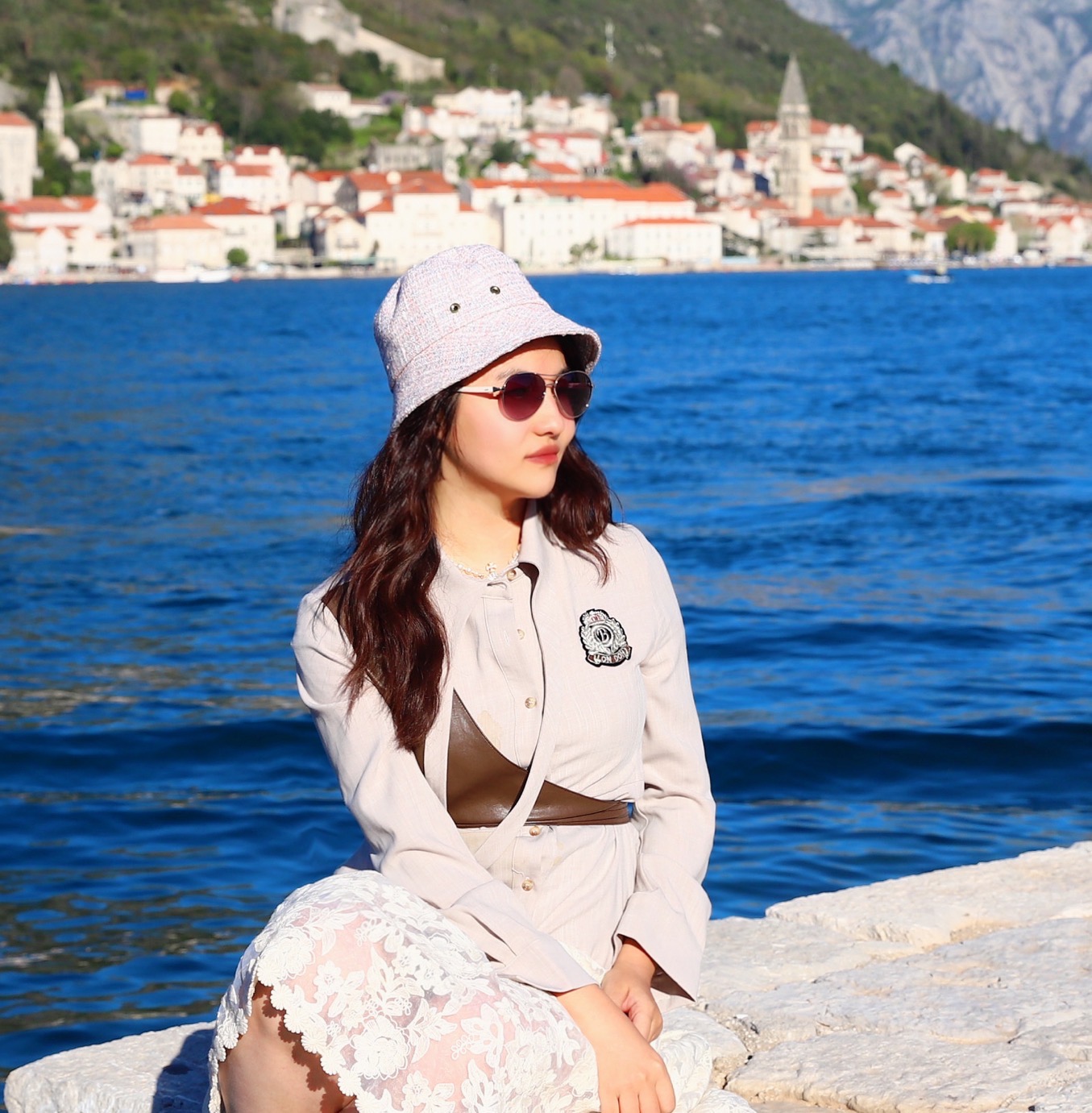
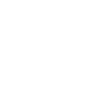
by Siqi Tian
As someone also deeply entrenched in the fields of autonomous driving and artificial intelligence, how does a scholar dedicated to the study of robot mobility perceive the surge of embodied intelligence in 2024?
At the World Robot Conference in Beijing, Jazzyear sat down with Wolfram Burgard, Director of the Laboratory for Robotics and Artificial Intelligence at the University of Technology Nuremberg. Professor Burgard is a highly respected figure in the academic world, renowned for his innovative probabilistic models that have significantly impacted robotic navigation and control systems, including localization, mapping, SLAM, and path planning. In 2009, Burgard was awarded the Leibniz Prize, Germany’s most prestigious research award.
In addition to his academic achievements, Burgard is the co-author of the seminal textbooks Probabilistic Robotics and Principles of Robot Motion: Theory, Algorithms, and Implementations. He also served as President of the IEEE Robotics and Automation Society. In the 2020 list of the world’s 2000 most influential AI scholars released by Tsinghua University, Burgard ranked first in the robotics category.
During our conversation, Burgard expressed his excitement about the advances in robotics driven by AI. However, he also highlighted that manufacturers often underestimate the perceptual capabilities required to truly achieve embodied intelligence. To genuinely understand the limitations of these systems, he suggests that sometimes the best approach is to put ourselves in the robot’s shoes—imagining ourselves with stiff hands and limited sensor capabilities.
He also believes that large language models, like ChatGPT, could enhance the explainability of robots. Although these models are not yet 100% accurate, “research shows that humans themselves are not always accurate in explaining things, and we are far from perfect in this regard. Therefore, “maybe we should lower our standards in that with regard as well. ” Burgard noted.
1. Underestimating the Perceptual Needs for Embodied Intelligence
Jazzyear: This is your third time attending the World Robot Conference. This year, there’s a noticeable presence of humanoid robots, and embodied intelligence has become a buzzword in 2024. As a roboticist, how do you view this trend?
Burgard: First of all, it becomes a little bit more clear what the application range of humanoids is and what these platforms could do in the future, particularly in production settings. On top of this, I see an enormous advancement in hardware, where the sheer number and quality of genuinely capable humanoid robots are impressive. I haven’t seen anything like this in Europe; in fact, there are not that many companies who are actually building robots of that performance.
A few years ago, robots at these exhibitions had to be tethered to the ceiling by ropes, with a student standing by to prevent them from malfunctioning. That was the state of the art in this technology. Now, we have numerous robots that can walk around without human supervision—this represents a significant leap forward. These robots demonstrate greater resilience and stability in the face of failures. A robot capable of performing continuous backflips was unimaginable just a few years ago.
Now, we have more reliable robots that meet the research needs of both academic and industrial laboratories. Once these robots are fully prepared for academic and industrial use, I believe we will see even more significant breakthroughs and developments in this field.
Jazzyear: But I noticed that many robots at the exhibition still require a rope on their back and need human guidance. What do you think is the most challenging technology for robots today?
Burgard: I agree that our robots still have ropes, but we are getting away from this.
Another challenge when it comes to the development of such robots lies in the perception that we need in the body to actually establish embodiment. Currently, these embodied intelligence platforms mostly rely on cameras, LiDAR, and sensors. While they can perceive the world, they still lack crucial sensors, such as those for touch and force.
To truly manipulate the world, robots need to understand how you grasp an object and whether they can securely hold something they’ve never picked up before. Humans can do this intuitively, so the current gap for robots is in their ability to judge force, which involves suitable hands and the necessary cables and computational power to relay information from the hands to the computer.
Jazzyear: With AI-driven technological breakthroughs, how has research in the robotics field changed?
Burgard: About two years ago, with the advent of large language models the public came aware of a breakthrough in AI. These models have opened up entirely new development spaces for AI. They give the impression of having a certain understanding of the real world.
For example, imagine you’re a robot, and your owner asks you to pick up a doll lying on the ground. In this case, if you ask a large language model like ChatGPT how to proceed, it might provide you with something similar to the following: first, identify the doll’s torso, then the arms and legs, and finally, suggest avoiding directly grabbing the head. This understanding of objects and how they should be manipulated was something that previously required complex programming and training to achieve.
Similarly, if a vacuum-cleaning robot has to decide whether to avoid or clean up an object in front of it, a large language model could provide guidance based on the object’s nature. For example, it might recommend avoiding jewelry or other valuable items while advising the cleaning of crumbs or other debris.
The intelligence of these large language models lies in their ability to perform tasks in a zero-shot fashion, without specific task training. This significantly reduces the need for manual programming, deep network training, and data collection. Thus, these models not only enhance the flexibility and adaptability of intelligent robots but also enable them to perform a wider variety of tasks in both household and production environments.
Jazzyear: Do you think robots should have legs so they can take on more human tasks at home?
Burgard: That’s a good question. I believe that removing wheels doesn’t necessarily make the problem easier. When you have legs, you can climb stairs and occupy a smaller footprint. However, this introduces greater instability—less force, reduced battery capacity, and diminished computing power. Overall, it’s a trade-off. I d think legs are necessary in all situations, but if a task can be accomplished with legs, that’s fine too.
Jazzyear: The Robot Conference showcased some humanoid robots that can do household chores, such as washing dishes and folding clothes. Do you think we will have household robots in the future? If the price is around $20,000, as Elon Musk suggested, would you buy one?
Burgard: If it’s useful, I would definitely buy one. In fact, I already have a lawn-mowing robot at home. I used to mow the lawn two to three times a week during the summer, each time for about half an hour. But now, I haven’t done it for years, and the lawn looks much better.
Jazzyear: I imagine hiring a human to mow your lawn would be much more expensive.
Burgard: Exactly. And in German society, there aren’t many people available to help with such tasks. Saving time is definitely an advantage. Of course, mowing the lawn is a physical activity that also provides exercise. If people spend their free time sitting idly, it could negatively impact their health. But in a household setting, a robot that automatically cleans the kitchen after breakfast would undoubtedly improve the quality of life.
However, the cost of robots is a significant challenge. The robots we see at exhibitions are prohibitively expensive, making them difficult to popularize. Additionally, although the hardware may be advanced, their intelligence still has room for improvement. Developing software on top of this advanced hardware requires even greater investment. The financial input for developing these robots, including software, could reach hundreds of millions of dollars. The cost of creating a flexible and practical household robot is very high.
Jazzyear: Another issue is that neural networks or large language models often lack explainability, creating a “black box.” This could pose significant risks for robots taking on daily household responsibilities, wouldn’t it?
Burgard: Certainly. As technology advances, our expectations of robots continue to rise. I was just discussing Asimov’s Laws of Robotics with some colleagues, which emphasize that robots should always serve humans and protect themselves without harming humans. However, what exactly does it mean to “harm a human”?
With large language models, we might be able to answer if a particular action would harm a human. Although we can’t fully achieve this yet, these models might provide up to 90% or more accuracy, which is undoubtedly a step forward. Explainability is indeed an issue, but if a robot’s performance surpasses that of a human, it might not need to explain its actions.
Jazzyear: That’s an interesting perspective. I think explainability also applies to autonomous driving. When autonomous systems lack explainability, they could pose significant risks. In this case, do you still believe that as long as a self-driving car performs better than a human, the lack of explainability isn’t a major issue?
Burgard: If robots could explain their actions, that would indeed be a significant advantage. Often, humans learn by understanding the reasoning behind actions. When learning tennis, for example, we seek out experts who can articulate why they make certain moves. This ability to explain could be seen as a powerful tool. However, research shows that humans are not always accurate in their explanations, and we, too, are far from perfect in this regard. Should we then accept that the standard for explanations, even by robots, might not need to be as high as we think?
When we ask a foundational model why a robot took a certain action, it might provide an explanation, though this explanation could simply be a story the model has generated. In some ways, we might be crafting a narrative to rationalize the robot’s behavior, but at the very least, this represents a step toward deeper understanding.
2.Observe the uncertainty in a robot perspective
Jazzyear: Let’s delve into your early years—what drew you to the field of robotics?
Burgard: I was captivated by the idea that robots, as physical agents in the world, could act according to my programming. This model fascinated me because it was the one domain where I could directly observe the outcomes of changes I made in code. Robotics was the only area where the results were immediately visible.
Jazzyear: How does the robotics industry and research landscape in Europe compare to that in China and the United States?
Burgard: I think China and the U.S. are more aggressive in their approach. There is a palpable enthusiasm for technological advancement, and significant investments are made once a technology matures and its applications become apparent. In contrast, Europeans tend to be more pessimistic and reluctant, often waiting for things to become more evident. Although European engineers are highly skilled, catching up in areas like embodied intelligence and hardware development will be a significant challenge. We need to respond more quickly to these advancements.
Jazzyear: It seems that Europeans excel in concepts, theories, and foundational research, while companies in the U.S. and China are more adept at commercializing these outcomes.
Burgard: That’s true. But on the other hand, our German engineers, and indeed engineers across Europe, have produced outstanding machinery in many industries. This is a significant advantage that shouldn’t be underestimated. However, I hope that Europe will become more receptive to new technologies and adopt a more optimistic and open attitude toward innovation, much like what I’ve observed in China.
Jazzyear: In your 2005 co-authored textbook Probabilistic Robotics, you wrote that the world is full of uncertainty from a robot’s perspective, which is why we study probability. How should we interpret this?
Burgard: Uncertainty is an enduring challenge in technology development. Even the most advanced foundational models cannot entirely eliminate system failures. Therefore, it is crucial to continuously measure uncertainty. Humans are not particularly adept at handling uncertainty either. For example, when driving in adverse weather, people often underestimate the risks and drive too fast, reflecting our inadequacy in managing uncertainty.
In robotics, we cannot ignore these challenges. Although deep networks perform exceptionally well on specific datasets, the complexity of the real world often exceeds their capabilities. Sensor failures and environmental unpredictability can significantly impact a robot’s performance.
Jazzyear: If we were to see the world from a robot’s perspective, what should we focus on, aside from uncertainty?
Burgard: Imagine being a robot equipped with its sensors and mechanical limbs, tasked with performing the same duties as a human. It’s far from easy. In fact, it’s a genuine challenge for robots.
Current robots, despite being able to execute many tasks, still have limitations in their perception abilities. To truly understand these systems’ limitations, sometimes the best approach is to put ourselves in the robot’s shoes—imagine possessing those less flexible hands and limited sensory capabilities—and then attempt to complete tasks. This experience will reveal that even simple tasks can become extraordinarily difficult in the robot’s world.
Jazzyear: I believe what sets humans apart is our accumulated common sense from life experiences, something that’s hard to impart to AI or robots.
Burgard: We expect foundational models to carry some basic common sense, though they may occasionally produce incorrect results, especially when handling complex calculations or arriving at unusual conclusions. However, when it comes to common-sense questions, these models often provide reasonable answers. For example, when asked about the amount of force needed to pick up an egg, ChatGPT can offer a specific value.
Jazzyear: What are your predictions for the development of robotics in the next five to ten years? What can we look forward to?
Burgard: Autonomous vehicles operating within safety frameworks are already being deployed in some cities and regions, and unmanned systems are gradually advancing. Over the next five to ten years, we can expect these technologies to see broader applications, particularly in factory settings where human-robot collaboration will become more commonplace. However, building a robust perception system requires significant time and resources, and the investment needed cannot be overlooked. Based on past developments, constructing such a system could take more than a decade.
In the industrial sector, where the environment is more controlled, standardized, and adaptable, robotic applications may be easier to implement. However, in household settings, where environments are more complex and unpredictable, robots will face greater challenges.
Jazzyear: Factories already have highly mature automation systems. Do we still need humanoid robots in such environments?
Burgard: In some cases, the challenge lies in dealing with enclosed spaces or objects with complex structures. Take aircraft assembly, for example—a task that is incredibly challenging. Unlike flat surfaces in open spaces, aircraft assembly involves complex curves and obstacles. In such environments, robots must be capable of overcoming obstacles and performing delicate operations within them.
Aircraft are designed and manufactured to accommodate human physiology and operational habits, which limits the applicability of robots in this field. If robots cannot handle aircraft assembly, we could face the dilemma of being unable to use them in this context. However, this is precisely where humanoid robots could demonstrate their potential. They may be able to carry out other similar construction tasks originally designed for humans.
Jazzyear: Could you reveal some of your upcoming research directions?
Burgard: That’s a great question. As scientists, we have a responsibility to imagine what the future holds. The probabilistic robotics approach we introduced marked a significant advancement, sparking a revolution in localization systems. This approach enabled robots to accurately determine their position in the world and build environmental maps, which were crucial in advancing autonomous vehicles. But we’re still not robust enough in this area.
This is where deep networks come into play. They offer a powerful tool to help robots better perceive and understand their surroundings. As foundational models evolve, we are opening a new dimension where robots can gain a deeper understanding of the world. The integration and application of these models could enable robots to perform tasks without relying entirely on traditional probabilistic robotics theory. The main challenge lies in how to effectively combine these technologies to form a cohesive system.
Jazzyear: After decades of research in robotics, has your belief in the field changed?
Burgard: Not at all. This field has seen tremendous progress, from sensors to humanoid robots. I believe we will see real humanoid robots within the next five to ten years. Yet, the field remains as captivating as ever.
-
25498
-
302
-
0
-
0